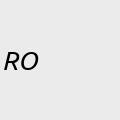
2024-04-15 08:35:51
This https://arxiv.org/abs/2404.05582 has been replaced.
initial toot: https://mastoxiv.page/@arXiv_csRO_…
This https://arxiv.org/abs/2404.05582 has been replaced.
initial toot: https://mastoxiv.page/@arXiv_csRO_…
Function Class Learning with Genetic Programming: Towards Explainable Meta Learning for Tumor Growth Functionals
E. M. C. Sijben, J. C. Jansen, P. A. N. Bosman, T. Alderliesten
https://arxiv.org/abs/2402.12510 https://arxiv.org/pdf/2402.12510
arXiv:2402.12510v1 Announce Type: new
Abstract: Paragangliomas are rare, primarily slow-growing tumors for which the underlying growth pattern is unknown. Therefore, determining the best care for a patient is hard. Currently, if no significant tumor growth is observed, treatment is often delayed, as treatment itself is not without risk. However, by doing so, the risk of (irreversible) adverse effects due to tumor growth may increase. Being able to predict the growth accurately could assist in determining whether a patient will need treatment during their lifetime and, if so, the timing of this treatment. The aim of this work is to learn the general underlying growth pattern of paragangliomas from multiple tumor growth data sets, in which each data set contains a tumor's volume over time. To do so, we propose a novel approach based on genetic programming to learn a function class, i.e., a parameterized function that can be fit anew for each tumor. We do so in a unique, multi-modal, multi-objective fashion to find multiple potentially in- teresting function classes in a single run. We evaluate our approach on a synthetic and a real-world data set. By analyzing the resulting function classes, we can effectively explain the general patterns in the data.
Oracle's controversial stewardship of Java: The good and the bad.
Oracle is doing a good job in keeping Java relevant. But that's pretty much where it ends, says Azul CTO (free reg)
https://www.computing.co.uk/feature/4172927/or…
Function Class Learning with Genetic Programming: Towards Explainable Meta Learning for Tumor Growth Functionals
E. M. C. Sijben, J. C. Jansen, P. A. N. Bosman, T. Alderliesten
https://arxiv.org/abs/2402.12510 https://arxiv.org/pdf/2402.12510
arXiv:2402.12510v1 Announce Type: new
Abstract: Paragangliomas are rare, primarily slow-growing tumors for which the underlying growth pattern is unknown. Therefore, determining the best care for a patient is hard. Currently, if no significant tumor growth is observed, treatment is often delayed, as treatment itself is not without risk. However, by doing so, the risk of (irreversible) adverse effects due to tumor growth may increase. Being able to predict the growth accurately could assist in determining whether a patient will need treatment during their lifetime and, if so, the timing of this treatment. The aim of this work is to learn the general underlying growth pattern of paragangliomas from multiple tumor growth data sets, in which each data set contains a tumor's volume over time. To do so, we propose a novel approach based on genetic programming to learn a function class, i.e., a parameterized function that can be fit anew for each tumor. We do so in a unique, multi-modal, multi-objective fashion to find multiple potentially in- teresting function classes in a single run. We evaluate our approach on a synthetic and a real-world data set. By analyzing the resulting function classes, we can effectively explain the general patterns in the data.