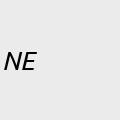
2024-02-23 06:51:16
A new approach for solving global optimization and engineering problems based on modified Sea Horse Optimizer
Fatma A. Hashim, Reham R. Mostafa, Ruba Abu Khurma, Raneem Qaddoura, P. A. Castillo
https://arxiv.org/abs/2402.14044 https://arxiv.org/pdf/2402.14044
arXiv:2402.14044v1 Announce Type: new
Abstract: Sea Horse Optimizer (SHO) is a noteworthy metaheuristic algorithm that emulates various intelligent behaviors exhibited by sea horses, encompassing feeding patterns, male reproductive strategies, and intricate movement patterns. To mimic the nuanced locomotion of sea horses, SHO integrates the logarithmic helical equation and Levy flight, effectively incorporating both random movements with substantial step sizes and refined local exploitation. Additionally, the utilization of Brownian motion facilitates a more comprehensive exploration of the search space. This study introduces a robust and high-performance variant of the SHO algorithm named mSHO. The enhancement primarily focuses on bolstering SHO's exploitation capabilities by replacing its original method with an innovative local search strategy encompassing three distinct steps: a neighborhood-based local search, a global non-neighbor-based search, and a method involving circumnavigation of the existing search region. These techniques improve mSHO algorithm's search capabilities, allowing it to navigate the search space and converge toward optimal solutions efficiently. The comprehensive results distinctly establish the supremacy and efficiency of the mSHO method as an exemplary tool for tackling an array of optimization quandaries. The results show that the proposed mSHO algorithm has a total rank of 1 for CEC'2020 test functions. In contrast, the mSHO achieved the best value for the engineering problems, recording a value of 0.012665, 2993.634, 0.01266, 1.724967, 263.8915, 0.032255, 58507.14, 1.339956, and 0.23524 for the pressure vessel design, speed reducer design, tension/compression spring, welded beam design, three-bar truss engineering design, industrial refrigeration system, multi-Product batch plant, cantilever beam problem, multiple disc clutch brake problems, respectively.