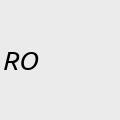
2024-05-02 08:30:21
This https://arxiv.org/abs/2404.19217 has been replaced.
initial toot: https://mastoxiv.page/@arXiv_csRO_…
This https://arxiv.org/abs/2404.19217 has been replaced.
initial toot: https://mastoxiv.page/@arXiv_csRO_…
An Effective Networks Intrusion Detection Approach Based on Hybrid Harris Hawks and Multi-Layer Perceptron
Moutaz Alazab, Ruba Abu Khurma, Pedro A. Castillo, Bilal Abu-Salih, Alejandro Martin, David Camacho
https://arxiv.org/abs/2402.14037 https://arxiv.org/pdf/2402.14037
arXiv:2402.14037v1 Announce Type: new
Abstract: This paper proposes an Intrusion Detection System (IDS) employing the Harris Hawks Optimization algorithm (HHO) to optimize Multilayer Perceptron learning by optimizing bias and weight parameters. HHO-MLP aims to select optimal parameters in its learning process to minimize intrusion detection errors in networks. HHO-MLP has been implemented using EvoloPy NN framework, an open-source Python tool specialized for training MLPs using evolutionary algorithms. For purposes of comparing the HHO model against other evolutionary methodologies currently available, specificity and sensitivity measures, accuracy measures, and mse and rmse measures have been calculated using KDD datasets. Experiments have demonstrated the HHO MLP method is effective at identifying malicious patterns. HHO-MLP has been tested against evolutionary algorithms like Butterfly Optimization Algorithm (BOA), Grasshopper Optimization Algorithms (GOA), and Black Widow Optimizations (BOW), with validation by Random Forest (RF), XG-Boost. HHO-MLP showed superior performance by attaining top scores with accuracy rate of 93.17%, sensitivity level of 89.25%, and specificity percentage of 95.41%.
This https://arxiv.org/abs/2306.06743 has been replaced.
initial toot: https://mastoxiv.page/@arXiv_hepe…
An Effective Networks Intrusion Detection Approach Based on Hybrid Harris Hawks and Multi-Layer Perceptron
Moutaz Alazab, Ruba Abu Khurma, Pedro A. Castillo, Bilal Abu-Salih, Alejandro Martin, David Camacho
https://arxiv.org/abs/2402.14037 https://arxiv.org/pdf/2402.14037
arXiv:2402.14037v1 Announce Type: new
Abstract: This paper proposes an Intrusion Detection System (IDS) employing the Harris Hawks Optimization algorithm (HHO) to optimize Multilayer Perceptron learning by optimizing bias and weight parameters. HHO-MLP aims to select optimal parameters in its learning process to minimize intrusion detection errors in networks. HHO-MLP has been implemented using EvoloPy NN framework, an open-source Python tool specialized for training MLPs using evolutionary algorithms. For purposes of comparing the HHO model against other evolutionary methodologies currently available, specificity and sensitivity measures, accuracy measures, and mse and rmse measures have been calculated using KDD datasets. Experiments have demonstrated the HHO MLP method is effective at identifying malicious patterns. HHO-MLP has been tested against evolutionary algorithms like Butterfly Optimization Algorithm (BOA), Grasshopper Optimization Algorithms (GOA), and Black Widow Optimizations (BOW), with validation by Random Forest (RF), XG-Boost. HHO-MLP showed superior performance by attaining top scores with accuracy rate of 93.17%, sensitivity level of 89.25%, and specificity percentage of 95.41%.
This https://arxiv.org/abs/2305.17799 has been replaced.
link: https://scholar.google.com/scholar?q=a
BMLP: Behavior-aware MLP for Heterogeneous Sequential Recommendation
Weixin Li, Yuhao Wu, Yang Liu, Weike Pan, Zhong Ming
https://arxiv.org/abs/2402.12733 …
A Comparison of Traditional and Deep Learning Methods for Parameter Estimation of the Ornstein-Uhlenbeck Process
Jacob Fein-Ashley
https://arxiv.org/abs/2404.11526
This https://arxiv.org/abs/2309.06067 has been replaced.
initial toot: https://mastoxiv.page/@arXiv_ees…