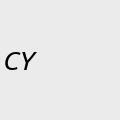
2024-06-13 07:23:41
Supporting Self-Reflection at Scale with Large Language Models: Insights from Randomized Field Experiments in Classrooms
Harsh Kumar, Ruiwei Xiao, Benjamin Lawson, Ilya Musabirov, Jiakai Shi, Xinyuan Wang, Huayin Luo, Joseph Jay Williams, Anna Rafferty, John Stamper, Michael Liut
https://arxiv.org/abs/2406.07571 https://arxiv.org/pdf/2406.07571
arXiv:2406.07571v1 Announce Type: new
Abstract: Self-reflection on learning experiences constitutes a fundamental cognitive process, essential for the consolidation of knowledge and the enhancement of learning efficacy. However, traditional methods to facilitate reflection often face challenges in personalization, immediacy of feedback, engagement, and scalability. Integration of Large Language Models (LLMs) into the reflection process could mitigate these limitations. In this paper, we conducted two randomized field experiments in undergraduate computer science courses to investigate the potential of LLMs to help students engage in post-lesson reflection. In the first experiment (N=145), students completed a take-home assignment with the support of an LLM assistant; half of these students were then provided access to an LLM designed to facilitate self-reflection. The results indicated that the students assigned to LLM-guided reflection reported increased self-confidence and performed better on a subsequent exam two weeks later than their peers in the control condition. In the second experiment (N=112), we evaluated the impact of LLM-guided self-reflection against other scalable reflection methods, such as questionnaire-based activities and review of key lecture slides, after assignment. Our findings suggest that the students in the questionnaire and LLM-based reflection groups performed equally well and better than those who were only exposed to lecture slides, according to their scores on a proctored exam two weeks later on the same subject matter. These results underscore the utility of LLM-guided reflection and questionnaire-based activities in improving learning outcomes. Our work highlights that focusing solely on the accuracy of LLMs can overlook their potential to enhance metacognitive skills through practices such as self-reflection. We discuss the implications of our research for the Edtech community.