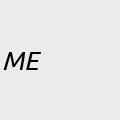
2024-03-01 07:20:46
On the Improvement of Predictive Modeling Using Bayesian Stacking and Posterior Predictive Checking
Mariana Nold, Florian Meinfelder, David Kaplan
https://arxiv.org/abs/2402.19046
On the Improvement of Predictive Modeling Using Bayesian Stacking and Posterior Predictive Checking
Mariana Nold, Florian Meinfelder, David Kaplan
https://arxiv.org/abs/2402.19046
If the world economy is back on track, it’s apparently a slower track.
Last month the World Bank released its updated report on Global Economic Prospects.
It drew a gloomy picture of slowing growth, marking what it calls a “wretched milestone” – a world economy that is expected to grow at its slowest rate in three decades:
2.4 per cent this year, with perhaps a slight improvement next year.
As to all that money sitting on the sidelines, it’s still sitting there.
Daily Inspiration: "World class innovators focus on long term wins through constant incremental improvements" - Futurist Jim Carroll
Want something boring? This is it! It's the BIG impact of the new SMALL incrementalism!
Huh what?
It involves thinking like this: “How can we continue to evolve our solutions in small ways that provide regular, continuous improvement for our customers?” And a realization that success can come from pursuing a mindset that success …
A good management system doesn’t rely on heroic efforts to save the day. The organization is designed to succeed. It is robust. It will succeed with all the variation thrown at it by the outside world. A good management system takes advantage of the contributions people offer, but it will not perform poorly when others are relied on.
A well run organization has graceful degradation (when one component fails or one person is missing...
Agile Requirement Change Management Model for Global Software Development
Neha Koulecar, Bachan Ghimire
https://arxiv.org/abs/2402.14595 https://
Brain-inspired Distributed Memorization Learning for Efficient Feature-free Unsupervised Domain Adaptation
Jianming Lv, Depin Liang, Zequan Liang, Yaobin Zhang, Sijun Xia
https://arxiv.org/abs/2402.14598 https://arxiv.org/pdf/2402.14598
arXiv:2402.14598v1 Announce Type: new
Abstract: Compared with gradient based artificial neural networks, biological neural networks usually show a more powerful generalization ability to quickly adapt to unknown environments without using any gradient back-propagation procedure. Inspired by the distributed memory mechanism of human brains, we propose a novel gradient-free Distributed Memorization Learning mechanism, namely DML, to support quick domain adaptation of transferred models. In particular, DML adopts randomly connected neurons to memorize the association of input signals, which are propagated as impulses, and makes the final decision by associating the distributed memories based on their confidence. More importantly, DML is able to perform reinforced memorization based on unlabeled data to quickly adapt to a new domain without heavy fine-tuning of deep features, which makes it very suitable for deploying on edge devices. Experiments based on four cross-domain real-world datasets show that DML can achieve superior performance of real-time domain adaptation compared with traditional gradient based MLP with more than 10% improvement of accuracy while reducing 87% of the timing cost of optimization.
Large Language Model with Graph Convolution for Recommendation
Yingpeng Du, Ziyan Wang, Zhu Sun, Haoyan Chua, Hongzhi Liu, Zhonghai Wu, Yining Ma, Jie Zhang, Youchen Sun
https://arxiv.org/abs/2402.08859
Brain-inspired Distributed Memorization Learning for Efficient Feature-free Unsupervised Domain Adaptation
Jianming Lv, Depin Liang, Zequan Liang, Yaobin Zhang, Sijun Xia
https://arxiv.org/abs/2402.14598 https://arxiv.org/pdf/2402.14598
arXiv:2402.14598v1 Announce Type: new
Abstract: Compared with gradient based artificial neural networks, biological neural networks usually show a more powerful generalization ability to quickly adapt to unknown environments without using any gradient back-propagation procedure. Inspired by the distributed memory mechanism of human brains, we propose a novel gradient-free Distributed Memorization Learning mechanism, namely DML, to support quick domain adaptation of transferred models. In particular, DML adopts randomly connected neurons to memorize the association of input signals, which are propagated as impulses, and makes the final decision by associating the distributed memories based on their confidence. More importantly, DML is able to perform reinforced memorization based on unlabeled data to quickly adapt to a new domain without heavy fine-tuning of deep features, which makes it very suitable for deploying on edge devices. Experiments based on four cross-domain real-world datasets show that DML can achieve superior performance of real-time domain adaptation compared with traditional gradient based MLP with more than 10% improvement of accuracy while reducing 87% of the timing cost of optimization.
Predicting Mergers and Acquisitions in Competitive Industries: A Model Based on Temporal Dynamics and Industry Networks
Dayu Yang
https://arxiv.org/abs/2404.07298 https://arxiv.org/pdf/2404.07298
arXiv:2404.07298v1 Announce Type: new
Abstract: M&A activities are pivotal for market consolidation, enabling firms to augment market power through strategic complementarities. Existing research often overlooks the peer effect, the mutual influence of M&A behaviors among firms, and fails to capture complex interdependencies within industry networks. Common approaches suffer from reliance on ad-hoc feature engineering, data truncation leading to significant information loss, reduced predictive accuracy, and challenges in real-world application. Additionally, the rarity of M&A events necessitates data rebalancing in conventional models, introducing bias and undermining prediction reliability. We propose an innovative M&A predictive model utilizing the Temporal Dynamic Industry Network (TDIN), leveraging temporal point processes and deep learning to adeptly capture industry-wide M&A dynamics. This model facilitates accurate, detailed deal-level predictions without arbitrary data manipulation or rebalancing, demonstrated through superior evaluation results from M&A cases between January 1997 and December 2020. Our approach marks a significant improvement over traditional models by providing detailed insights into M&A activities and strategic recommendations for specific firms.
Large Language Model with Graph Convolution for Recommendation
Yingpeng Du, Ziyan Wang, Zhu Sun, Haoyan Chua, Hongzhi Liu, Zhonghai Wu, Yining Ma, Jie Zhang, Youchen Sun
https://arxiv.org/abs/2402.08859
Double-stranded RNA activated caspase oligomerizer ( #DRACO ) technology, is an approach to ♦️selectively killing cells in which viral replication is taking place. ♦️
DRACO offered the promise of being broadly and rapidly effective for 🔸ending infection by many different viruses, and doing so with little adaptation of the core technology from virus to virus,🔸 a big improvement over the present state of…